What is a probability of default model?
Since 2009, more than 400 financial institutions have become insolvent. Each institution was determined by examiners to be ill-equipped to cover the unforeseen level of borrower defaults and resulting losses they had to recognize. The common sentiment amongst these banks – “if only we had seen this coming or had been better prepared…”
For this reason, many financial institutions are looking for a way to proactively identify and quantify the risks that they face. In that pursuit, institutions are devoting an unprecedented amount of time and resources towards developing or purchasing credit risk models that may enhance their abilities to predict and quantify the credit risks they face. These credit models can also enhance their abilities to calculate and assign sufficient economic capital reserves. These efforts have been recognized and promoted by bank regulators and their macroprudential policies.
Learn how to book loans faster while managing risk.
One such risk model, the probability of default model (PDM), is at the forefront of bankers’ and regulators’ minds. It is also used outside of banking to generally assess the creditworthiness of a company. A PDM helps a bank uniformly quantify credit risk – the predominant source of risk for banks and the subject of strict regulatory oversight.
The PDM assesses credit risk, the risk of a loss resulting from the failure of borrowers to meet payment obligations. The PDM estimates the likelihood that a particular loan or pool of loans will not be repaid and, thus, will fall into default within a specified time horizon. A probability of default model uses multivariate analysis and examines multiple characteristics or variables of the borrower, and it will usually account for credit or business cycles by either incorporating current financial data into the generation of the model or by including economic adjustments.
Each variable’s coefficient in the PDM provides management with an indication of the strength of correlation, whether positive or negative, between that variable and the occurrence of default. The higher the coefficient’s magnitude, the more significant of a relationship it has with default.
For example, the Altman Z-score is a model that was calibrated and published in 1968. The original model was
Z = 0.012T1 + 0.014T2 + 0.033T3 + 0.006T4 + 0.009T5
The variable T3 therefore is more predictive of a company’s default risk since it is the largest coefficient.
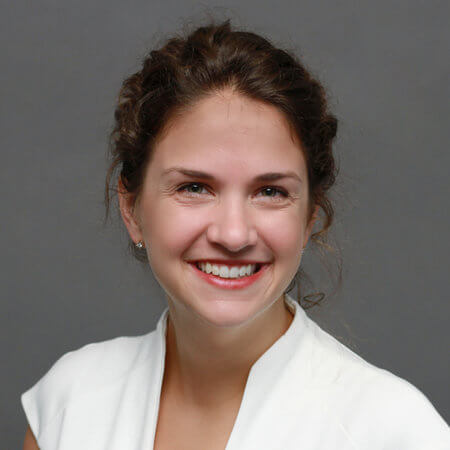