6 Common CECL backtesting mistakes & how to avoid them
April 14, 2025
Read Time: 0 min
About the Author
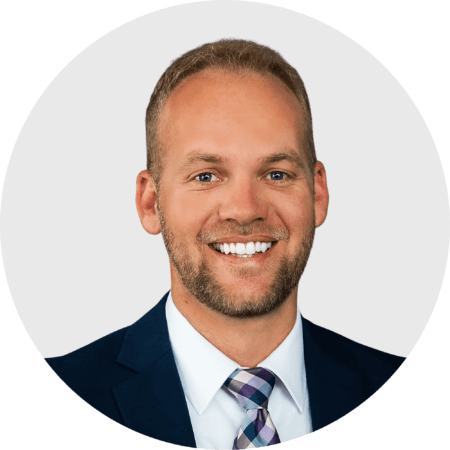
Abrigo enables U.S. financial institutions to support their communities through technology that fights financial crime, grows loans and deposits, and optimizes risk. Abrigo's platform centralizes the institution's data, creates a digital user experience, ensures compliance, and delivers efficiency for scale and profitable growth.
Make Big Things Happen.